Adding a wordcloud
Since the Basic Functions layer is now unbalanced, let’s add a beautiful wordcloud on the right, just to make everything much more symmetric. Please double-check that you already imported the Wordcloud library, as suggested in Chapter 4. This time, we can add another expander into the second column and write a few lines of code, as illustrated in the following figure:
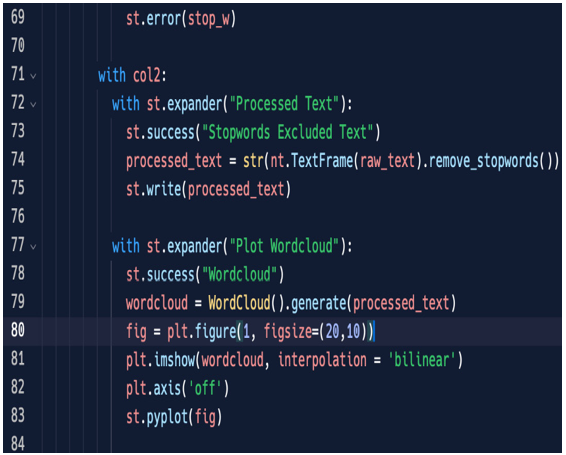
Figure 5.19: Wordcloud plotting code
A few lines of code and a wordcloud will appear! So, we add another expander, then create a wordcloud from the original text using the generate method, and then define a figure with its size. Finally, use plt (we already imported pyplot) to plot the wordcloud without axis. We used a longer original text for a richer wordcloud. The bigger the words appear, the more often they occur in the text. This is the result:
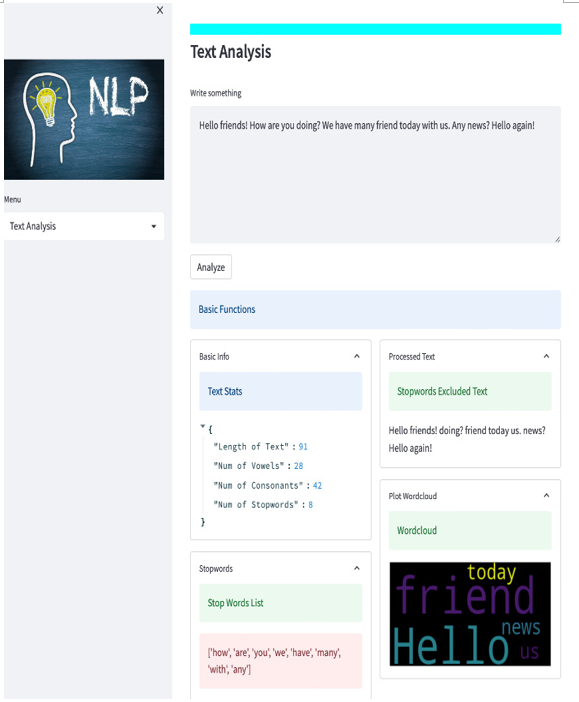
Figure 5.20: Wordcloud on the screen
Now our Basic Functions part really is finished. We have a beautiful text that shows the beginning of the section and two columns, and in each of them, two expanders, with everything working well and fluidly. It’s time to address the advanced features, such as handling tokens, lemmas, and summarization. We will discuss these in detail further in this chapter.
Introducing NLP concepts – tokens and lemmas
Let’s begin exploring Advanced Features by creating a simple summarization function in Python and Streamlit. Although many packages and libraries offer powerful summarization capabilities, this book focuses on web application development rather than NLP or summarization.
Adding the summarization function
Though the name is self-explanatory, a summarization function is a piece of code that summarizes a sentence or a text, extracting only the most important part of it. This task can be achieved in many ways – some very easy, like the one we are proposing just to show how to develop complex web applications with Streamlit, and some very sophisticated, leveraging artificial intelligence and neural networks. Figure 5.21 shows the code where we add the summarize_text function:
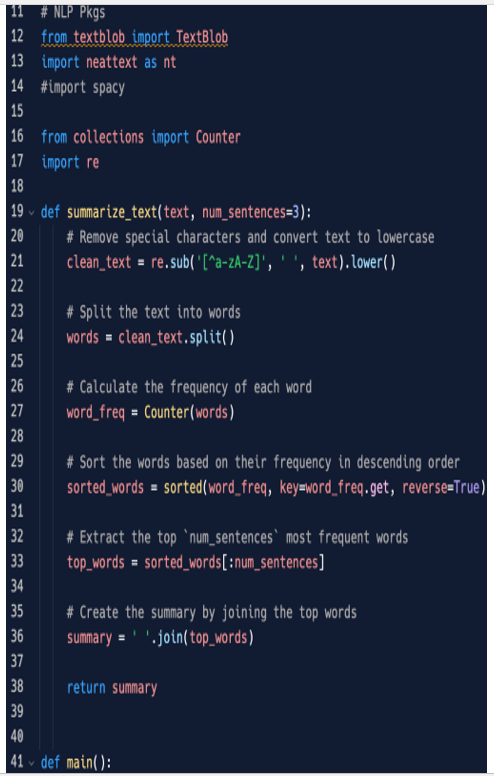
Figure 5.21: The summarize_text function
An attention point is that after the NLP packages, we imported two new libraries:
from collections import Counter
import re
Both of them are Python standard packages; the first one is a set of collections that includes a counter and the second is the regular expressions package.
After this import activity, we defined the summarize_text function by writing the following code:
def summarize_text(text, num_sentences=3):
This function is very simple: it takes text as input, cleans the text by lowercasing it, splits everything into words, calculates the frequency of each word (that’s why we need the counter), sorts the words according to their frequency, extracts the most frequent, and then creates a summary just by joining the top words.
This function can be used inside the expander of the summarization as follows:
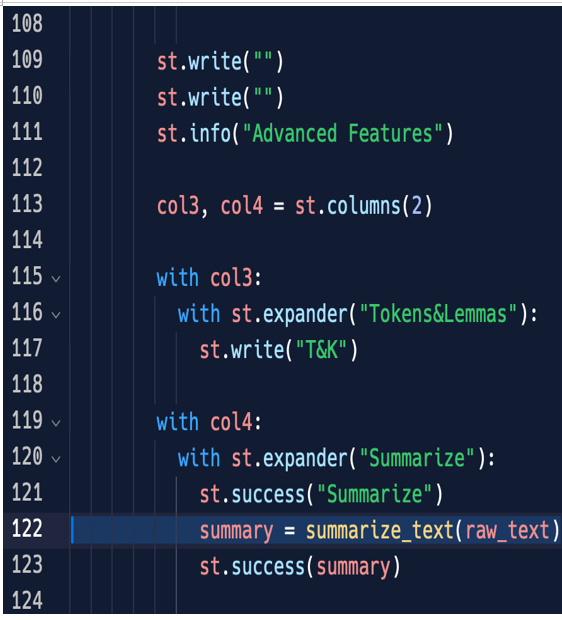
Figure 5.22: The Summarize expander
In the expander of col4, we are just using the summarize_text function with the input text (raw_text) and showing the result on the screen, as follows:
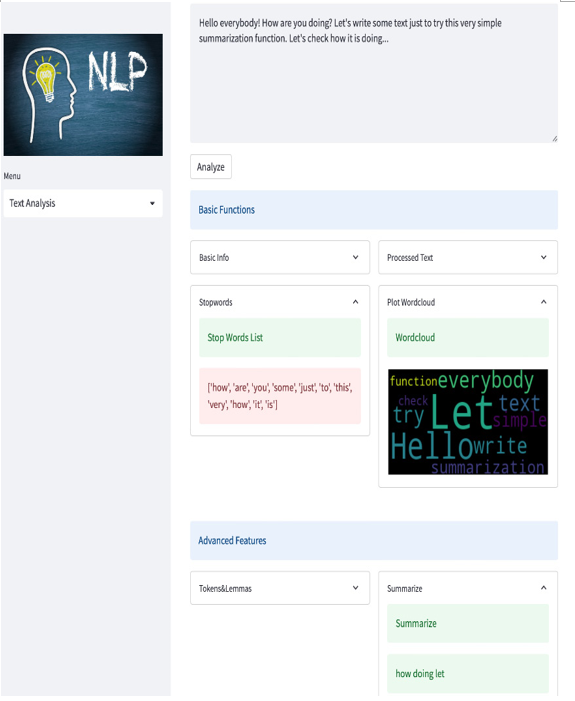
Figure 5.23: Summarize in action
OK, this feature is not the best, but why don’t you try to improve it by yourself? For example, you could add some advanced summarization features offered online by many companies via API calls.
Next, let us learn what tokens and lemmas are.